About CAMP
People understand the world by breaking down into parts. Events are perceived as a series of actions, objects are composed of multiple parts, and this sentence can be decomposed into a sequence of words. Although our knowledge representation is naturally compositional, most approaches to computer vision tasks generate representations that are not compositional.
We also understand that people use a variety of sensing modalities. Vision is an essential modality, but it can be noisy and requires a direct line of sight to perceive objects. Other sensors (e.g. audio, smell) can combat these shortcomings. They may allow us to detect otherwise imperceptible information about a scene. Prior workshops focused on multimodal learning have focused primarily on audio, video, and text as sensor modalities, but we found that these sensor modalities may not be inclusive enough.
Both these points present interesting components that can add structure to the task of activity/scene recognition yet appear to be underexplored. To help encourage further exploration in these areas, we believe a challenge with each of these aspects is appropriate.
We announce the 4th annual installment of the ”Compositionality and Multimodal Perception” Challenge (CAMP).
Dataset
Home Action Genome (HOMAGE)
Home Action Genome is a large-scale multi-view video database of indoor daily activities.
Every activity is captured by synchronized multi-view cameras, including an egocentric view.
Multi-Object Multi-Actor (MOMA)
The MOMA dataset is structured in a four-level hierarchy in terms of activity partonomy, with rich annotation at each level.
Program
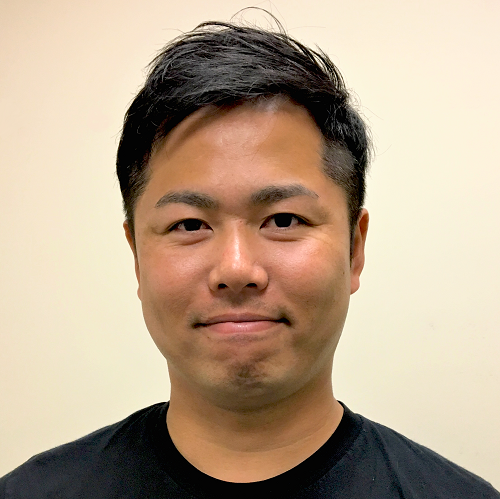
He is a manager at Panasonic Holdings Corporation. His research interests lie in visual understanding through machine learning, mainly for computer vision.
She is a second year Ph.D. student in Computer Science at the University of Washington under the guidance of Jeffrey Heer and Daniel Weld. As Artificial Intelligence systems increasingly impact daily life, I’m motivated to make the abilities and effects of these systems more interpretable. Drawing from my past work in Cognitive Science, Human-Computer Interaction, and Computer Vision, she is especially interested in framing these evaluations within a societal context and providing insights into how to improve model behavior and the decisions of Human-AI teams.
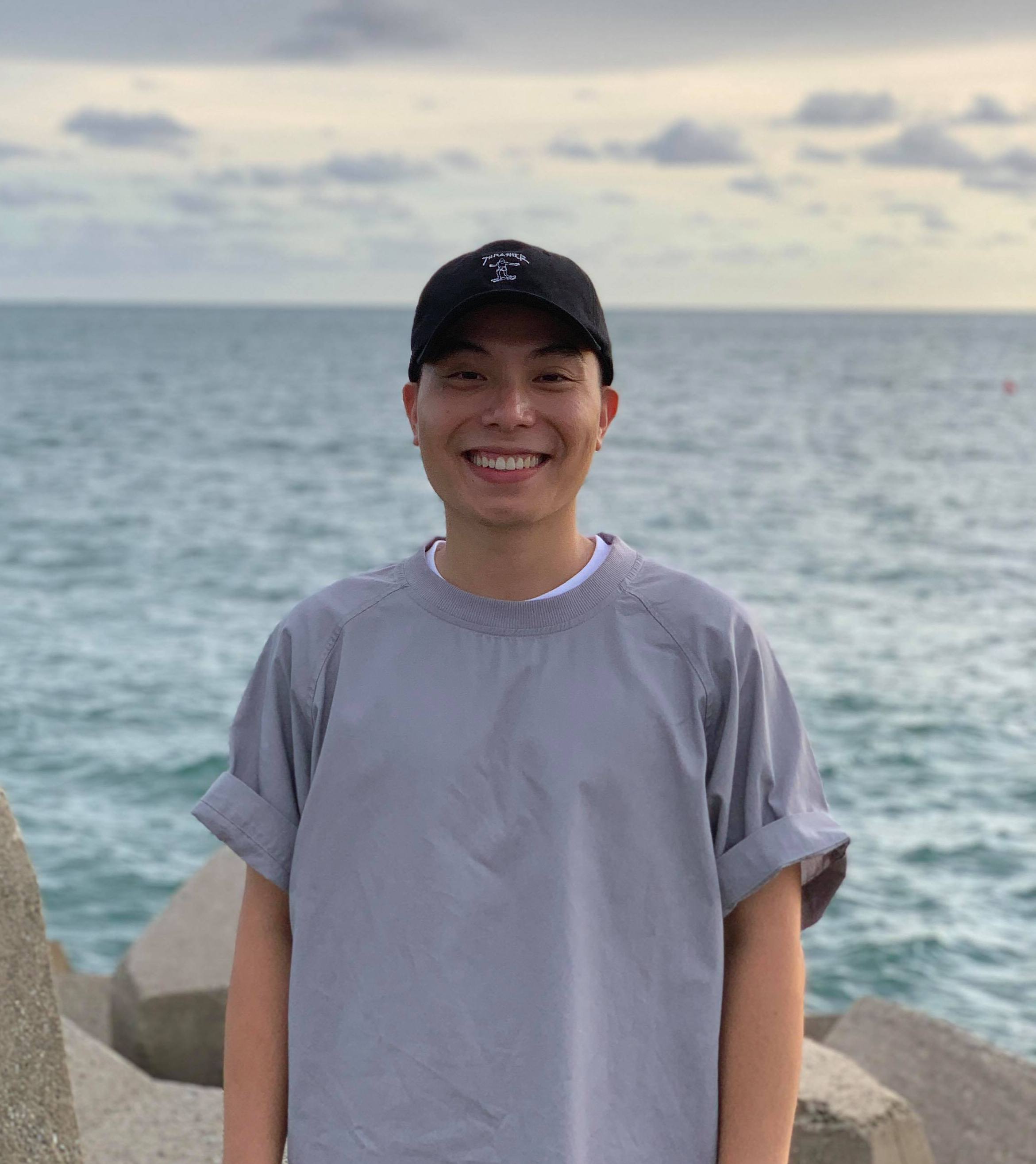
He is a Ph.D. student in Computer Science & Engineering at the University of Washington, working with Ranjay Krishna and Alex Ratner on tackling challenges in today’s large-scale machine learning environment. His research goal is to democratize AI development by making both data and model scaling more efficient and effective in today’s large-scale environment, based on four complementary areas of work tackling different aspects of data and model scaling challenges. On data side, he studies (1) how to efficiently curate large datasets, and (2) how to effectively align model behavior through data. On model side, he tackles (3) how to efficiently deploy large models, and (4) how to effectively adapt large models to downstream applications.
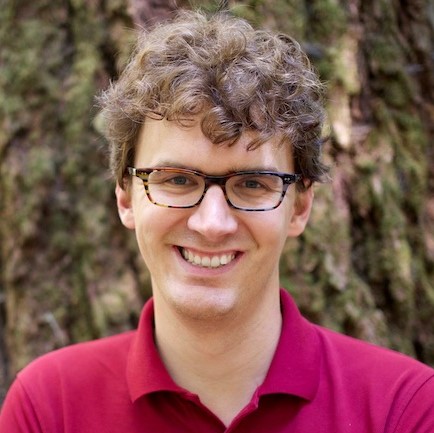
Carl Vondrick is an assistant professor of computer science at Columbia University. His research focuses on computer vision and machine learning. By training machines to observe and interact with their surroundings, we believe we can create robust and versatile models for perception. He often develop visual models that capitalize on large amounts of unlabeled data and transfer across tasks and modalities. Other interests include sound and language, interpretable models, high-level reasoning, and perception for robotics. Before Columbia, Carl was a research scientist at Google AI. He completed his PhD in computer science at the Massachusetts Institute of Technology in 2017, and his BS in computer science at the University of California, Irvine in 2011.
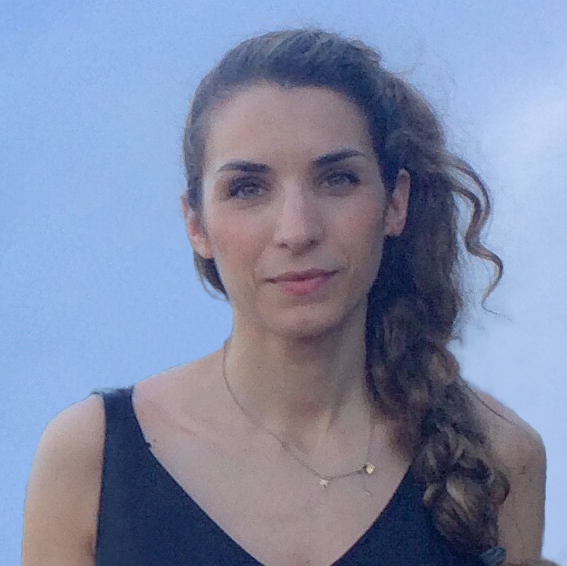
She is an assistant professor at the Department of Civil and Environmental Engineering, Stanford University, leading the Gradient Spaces group. She is interested in interdisciplinary research between Architecture, Civil Engineering, and Machine Perception. Her area of focus is on developing quantitative and data-driven methods that learn from real-world visual data to generate, predict, and simulate new or renewed built environments that place the human in the center. Her goal is to create sustainable, inclusive, and adaptive built environments that can support our current and future physical and digital needs.
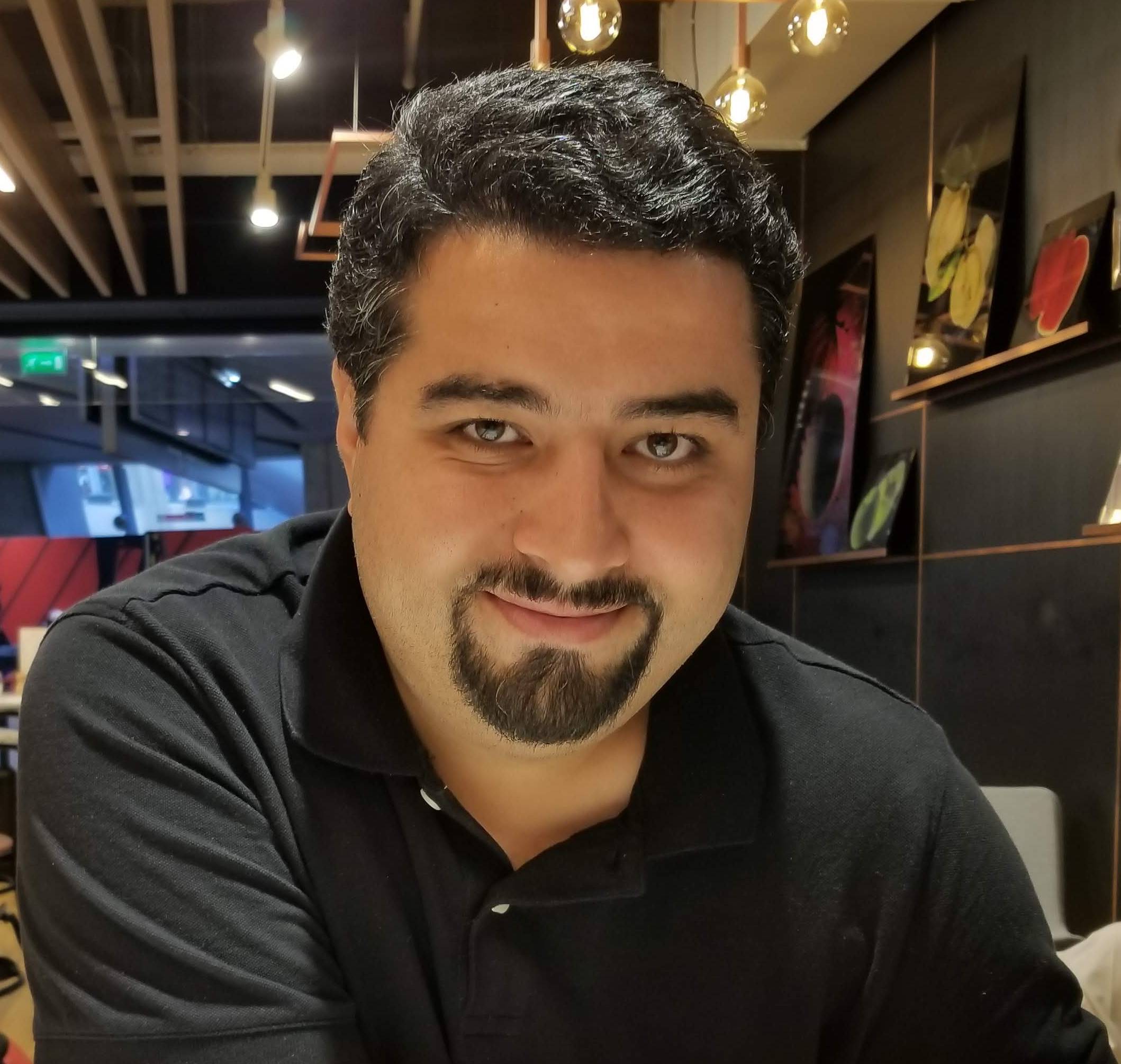
Dr. Ehsan Adeli is an assistant professor at Stanford University School of Medicine (Computational Neuroscience Lab) and is also affiliated with the Department of Computer Science at Stanford’s School of Engineering (Stanford Vision and Learning Lab). With a Ph.D. in computer vision and artificial intelligence, Dr. Adeli is applying his expertise to solve critical problems in healthcare and neuroscience.
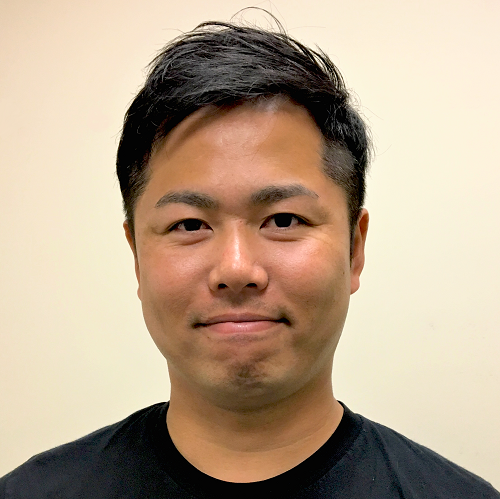
He is a manager at Panasonic Holdings Corporation. His research interests lie in visual understanding through machine learning, mainly for computer vision.
Organizers
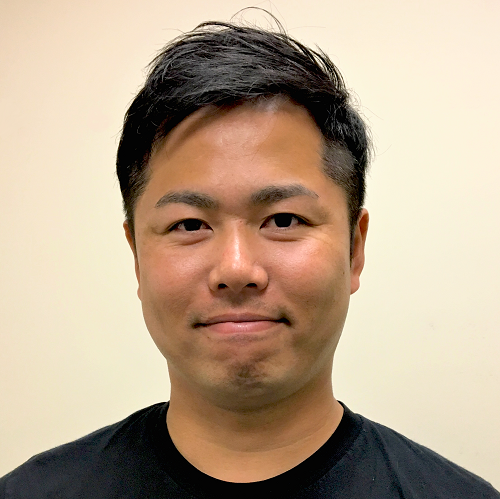
Kazuki Kozuka
Panasonic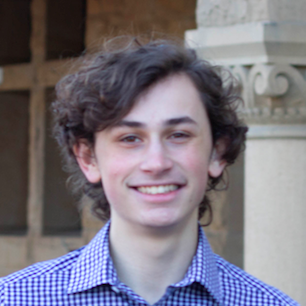
Edward Vendrow
Stanford University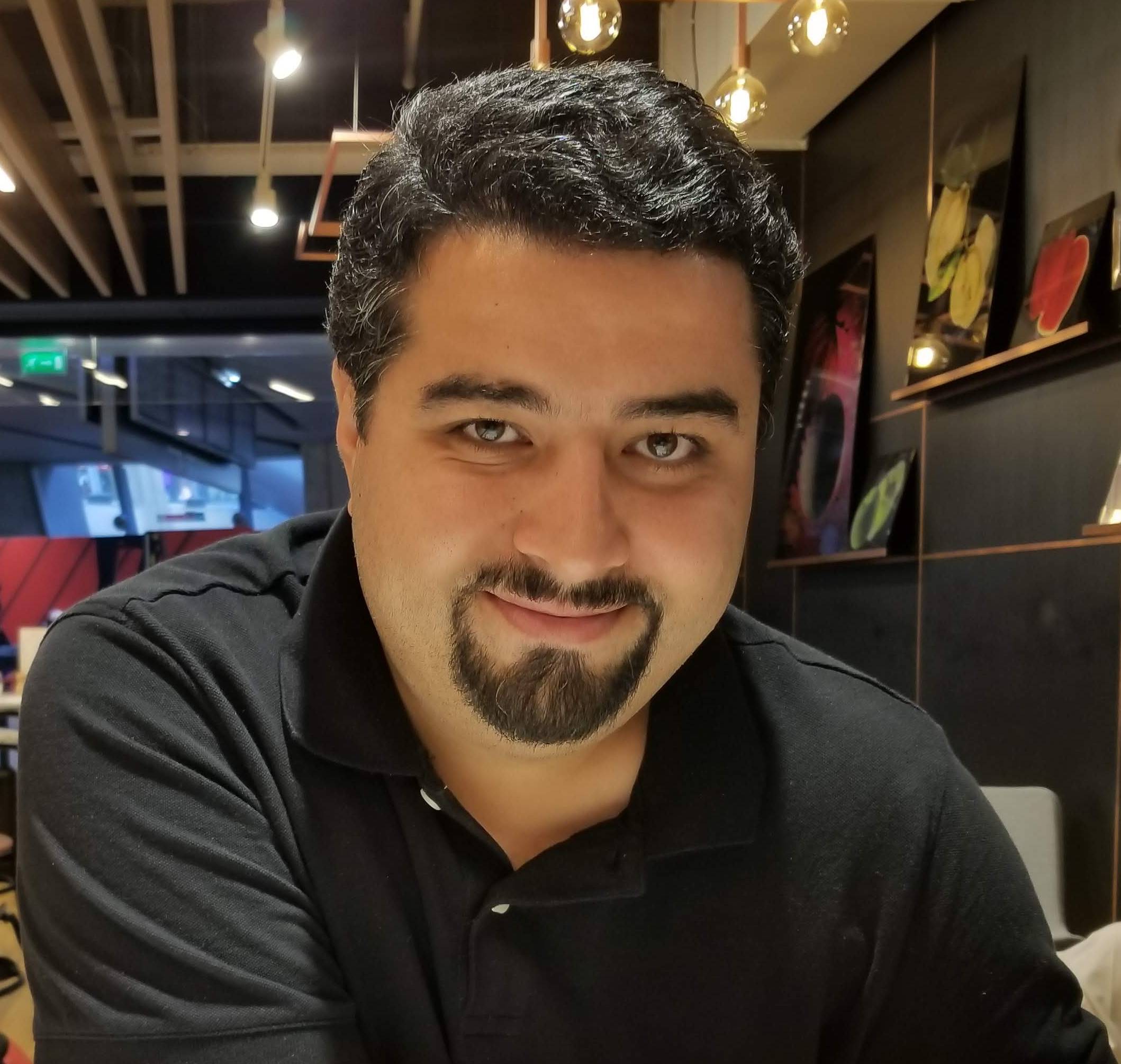
Ehsan Adeli
Stanford University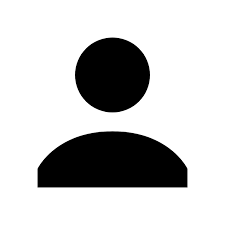
Jihoon Chung
Princeton UniversityOlga Russakovsky
Princeton UniversityMadeleine Grunde-McLaughin
University of Washington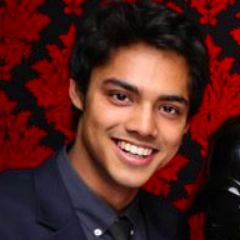
Ranjay Krishna
University of Washington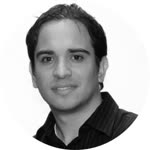
Juan Carlos Niebles
Salesforce Research, Stanford University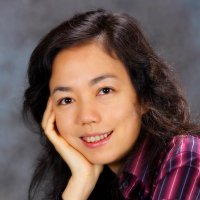
FeiFei Li
Stanford UniversityProgram Committees
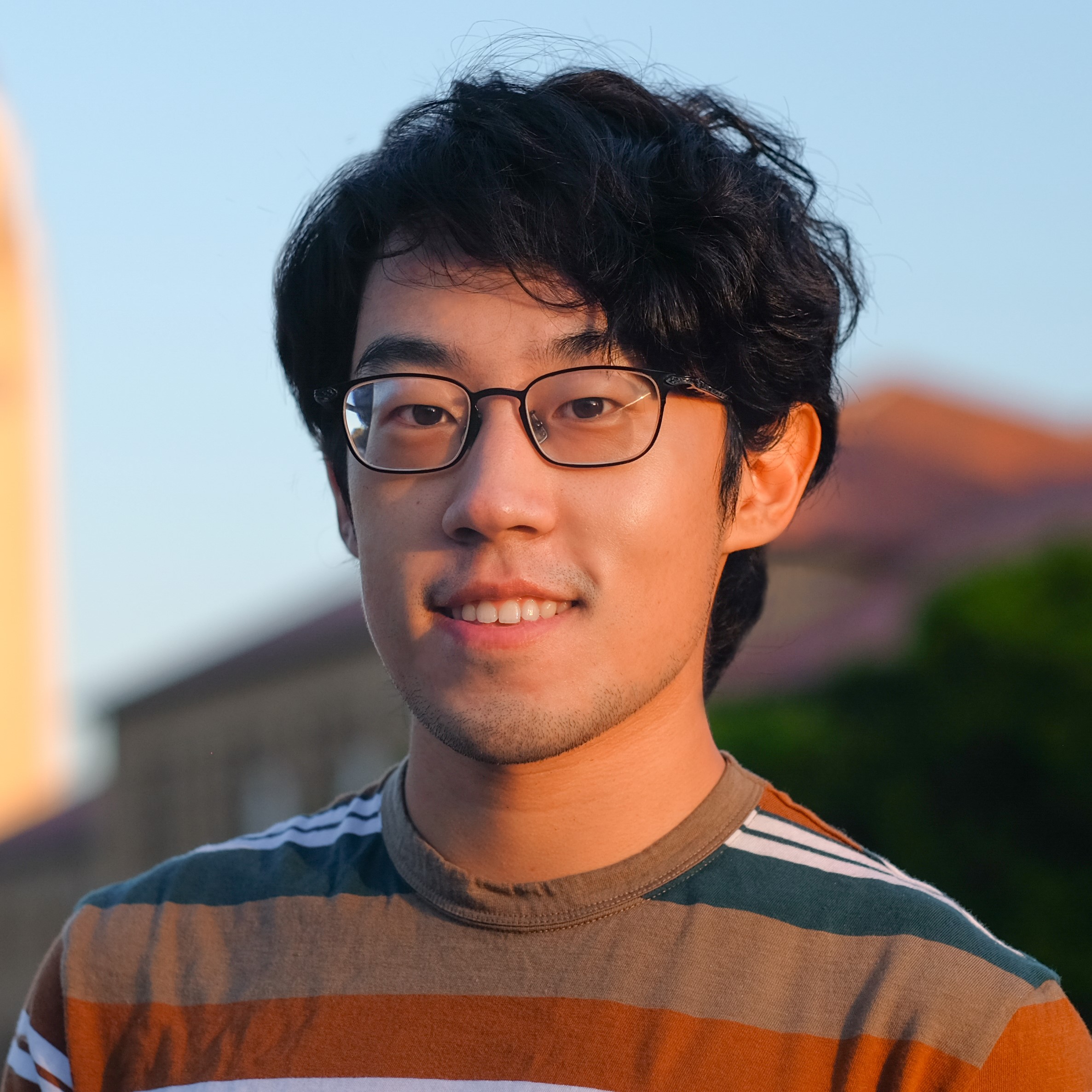
Haofeng Chen
Stanford University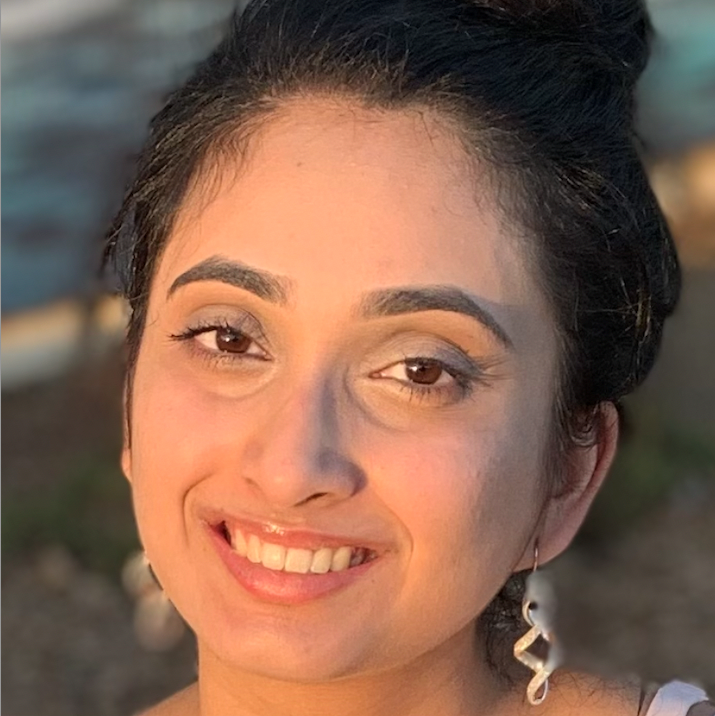
Neha Konakalla
Stanford University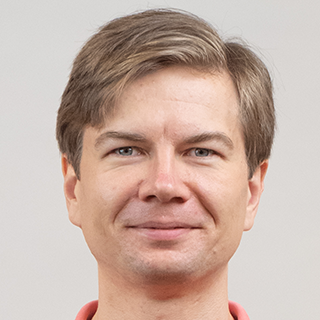
Denis Gudovskiy
Panasonic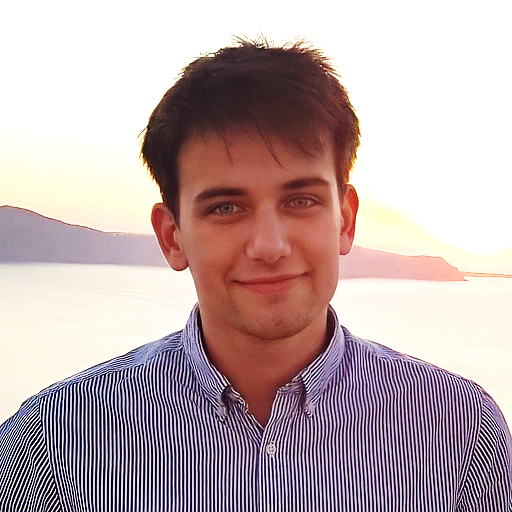
Konstantinos Kallidromitis
Panasonic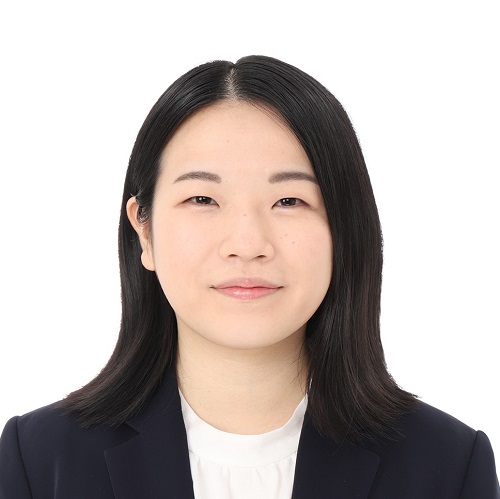
Risako Tanigawa
Panasonic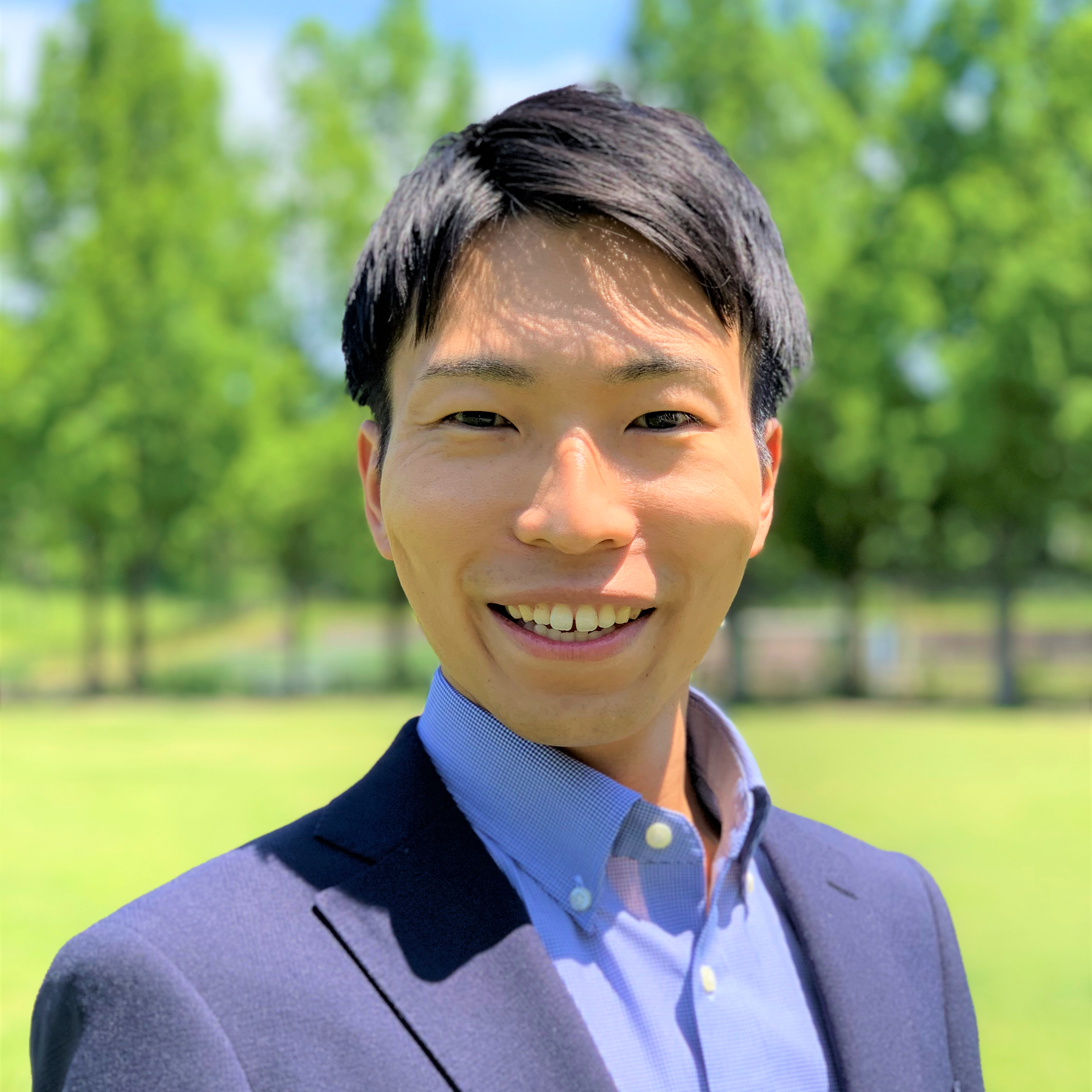